Abstract
Quantum neural networks (QNNs) play a pivotal role in addressing complex tasks within quantum machine learning, analogous to classical neural networks in deep learning. Ensuring consistent performance across diverse datasets is crucial for understanding and optimizing QNNs in both classical and quantum machine learning tasks, but remains a challenge as QNN’s generalization properties have not been fully explored. In this paper, we investigate the generalization properties of QNNs through the lens of learning algorithm stability, circumventing the need to explore the entire hypothesis space and providing insights into how classical optimizers influence QNN performance. By establishing a connection between QNNs and quantum combs, we examine the general behaviors of QNN models from a quantum information theory perspective. Leveraging the uniform stability of the stochastic gradient descent algorithm, we propose a generalization error bound determined by the number of trainable parameters, data uploading times, dataset dimension, and classical optimizer hyperparameters. Numerical experiments validate this comprehensive understanding of QNNs and align with our theoretical conclusions. As the first exploration into understanding the generalization capability of QNNs from a unified perspective of design and training, our work offers practical insights for applying QNNs in quantum machine learning.
Publication
arXiv:2501.16228
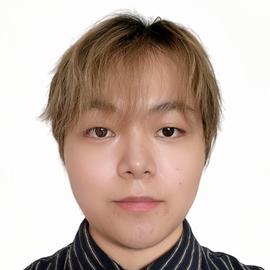
PhD Student (2023)
I obtained my BS and MS degrees in computer science from the University of Melbourne. My research interests include distributed quantum computing, quantum entanglement and quantum machine learning.
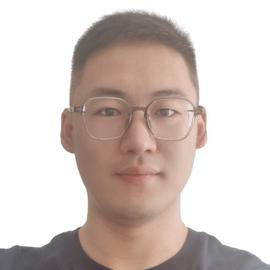
PhD Student (2024)
I obtained my BS degree in Mathematics from Nanjing University of Aeronautics and Astronautics and my MS degree in Mathematics from Beihang University. My research interests include quantum information theory and quantum machine learning.
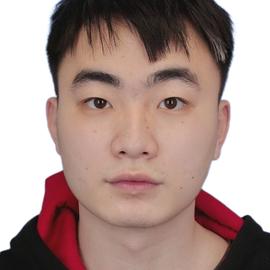
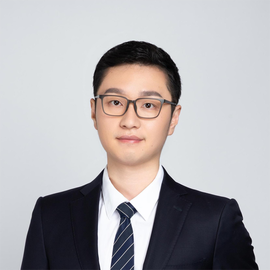
Associate Professor
Prof. Xin Wang founded the QuAIR lab at HKUST(Guangzhou) in June 2023. His research primarily focuses on better understanding the limits of information processing with quantum systems and the power of quantum artificial intelligence. Prior to establishing the QuAIR lab, Prof. Wang was a Staff Researcher at the Institute for Quantum Computing at Baidu Research, where he concentrated on quantum computing research and the development of the Baidu Quantum Platform. Notably, he spearheaded the development of Paddle Quantum, a Python library designed for quantum machine learning. From 2018 to 2019, Prof. Wang held the position of Hartree Postdoctoral Fellow at the Joint Center for Quantum Information and Computer Science (QuICS) at the University of Maryland, College Park. He earned his doctorate in quantum information from the University of Technology Sydney in 2018, under the guidance of Prof. Runyao Duan and Prof. Andreas Winter. In 2014, Prof. Wang obtained his B.S. in mathematics (with Wu Yuzhang Honor) from Sichuan University.