Paper accepted by NeurIPS 2023!
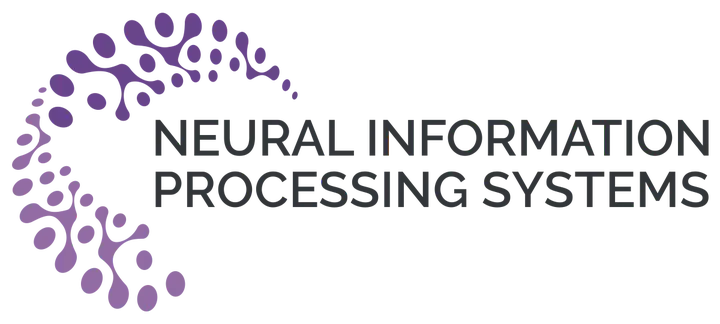
Paper on quantum neural network is accepted for NeurIPS 2023 🎉
Our paper Statistical Analysis of Quantum State Learning Process in Quantum Neural Networks by Haokai Zhang, Chenghong Zhu, Mingrui Jing and Xin Wang was accepted at NeurIPS 2023!
For NeurIPS 2023: The Thirty-Seventh Annual Conference on Neural Information Processing Systems (NeurIPS 2023) is an interdisciplinary conference that brings together researchers in machine learning, neuroscience, statistics, optimization, computer vision, natural language processing, life sciences, natural sciences, social sciences, and other adjacent fields.
For the paper Statistical Analysis of Quantum State Learning Process in Quantum Neural Networks: We develop a no-go theorem for learning an unknown quantum state with QNNs even starting from a high-fidelity initial state. We prove that when the loss value is lower than a critical threshold, the probability of avoiding local minima vanishes exponentially with the qubit count, while only grows polynomially with the circuit depth. The curvature of local minima is concentrated to the quantum Fisher information times a loss-dependent constant, which characterizes the sensibility of the output state with respect to parameters in QNNs. These results hold for any circuit structures, initialization strategies, and work for both fixed ansatzes and adaptive methods. Extensive numerical simulations are performed to validate our theoretical results. Our findings place generic limits on good initial guesses and adaptive methods for improving the learnability and scalability of QNNs, and deepen the understanding of prior information's role in QNNs.